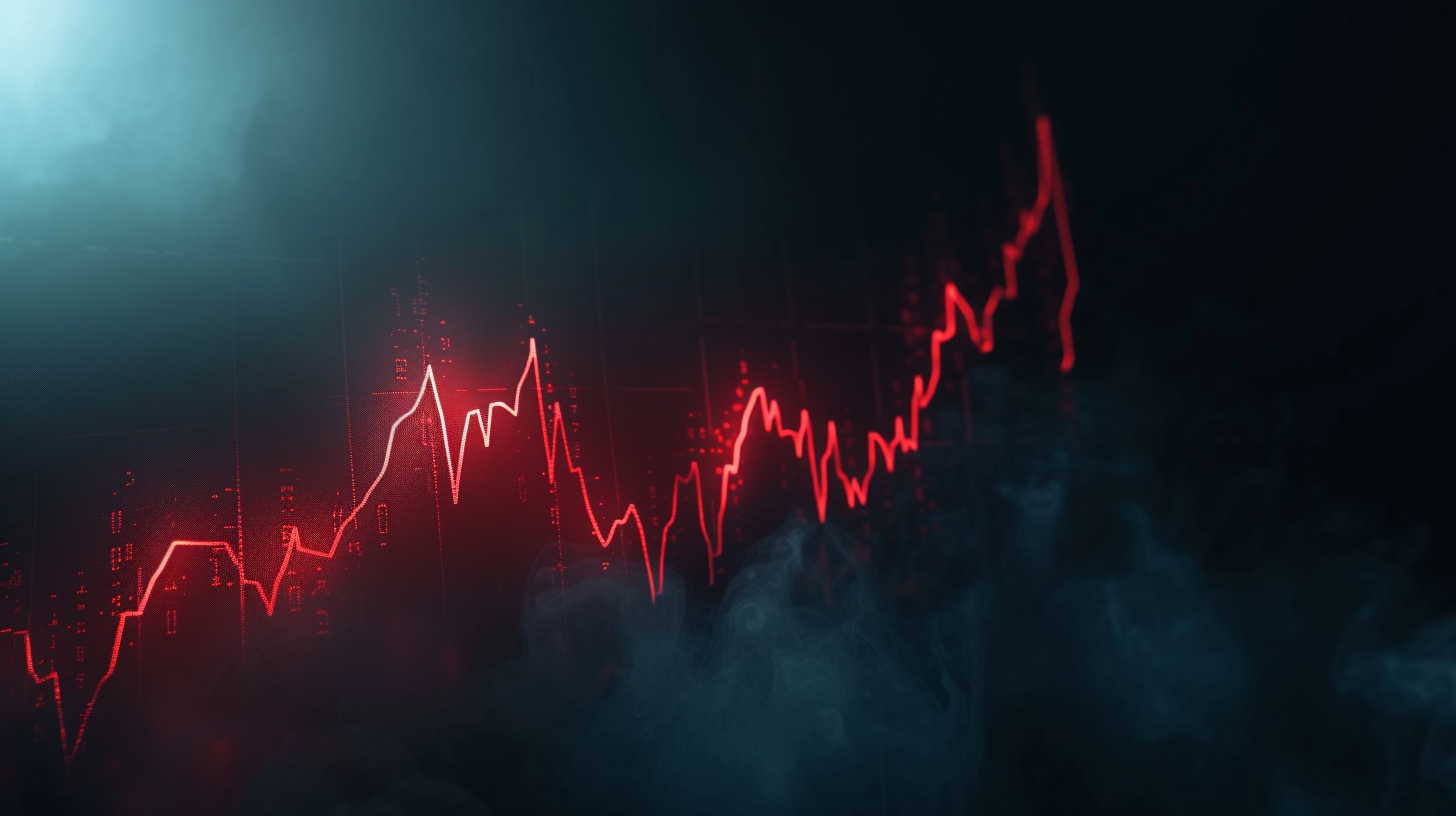
Stock Market Predication
Stock market predictions are forecasts about how stock prices will change in the future, based on analyzing past trends, market data, and economic indicators.
Introduction:
The stock market has always been unpredictable and volatile, with investors and traders
constantly seeking ways to forecast and predict future market movements. One popular
method used for stock market prediction is the application of machine learning algorithms. In
this case study, we explore the use of machine learning algorithms to predict stock market
movements.
Case Study:
A hedge fund specializing in trading stocks and derivatives tasked their team of traders with
developing a model to predict stock market movements with a high degree of accuracy. The
team decided to use machine-learning algorithms to develop a predictive model.
Data Collection and Preparation:
The first step in developing the predictive model was to collect and prepare data for analysis.
The team collected data on various stocks and indices from multiple sources, including
financial news websites and APIs from financial data providers.
The team also collected data on macroeconomic indicators such as GDP, inflation, and interest
rates. The team then cleaned and processed the data to remove any outliers and
inconsistencies.
Feature Engineering:
The team then created new features from the collected data to better represent the market’s
behaviour. The team created features such as moving averages, relative strength index (RSI),
and Bollinger bands, which are commonly used in technical analysis. The team also created
new features using natural language processing techniques to analyze financial news articles
and sentiment analysis to understand the market’s overall sentiment.
Model Development:
The team used several machine learning algorithms to develop the predictive model, including
random forest, gradient boosting, and neural networks. The team trained each algorithm on a
subset of the data and evaluated their performance on a test set. The team then selected the
algorithm with the best performance for deployment.
Model Deployment:
The team deployed the predictive model in a trading platform that automatically executed
trades based on the model’s predictions. The team also developed a dashboard that displayed
the model’s predictions and trading activity.
Results:
The model’s performance was evaluated using several metrics, including accuracy, precision,
and recall. The model achieved an accuracy of 66% in predicting the market’s direction. The
precision and recall were also high, indicating that the model correctly identified both positive
and negative market movements.
The hedge fund’s portfolio’s performance improved significantly after the deployment of the
predictive model. The fund’s management was pleased with the results and decided to allocate
more resources to further improve the model’s performance.
Conclusion:
In conclusion, the application of machine learning algorithms in stock market prediction has
shown promising results. The development of predictive models requires a deep understanding
of the market’s behaviour and the use of sophisticated data processing and modelling
techniques. However, the use of machine learning algorithms is not a silver bullet, and market
movements are still inherently unpredictable. Therefore, it is important to approach stock
market prediction with caution and to always monitor the model’s performance to ensure it
remains accurate and effective.
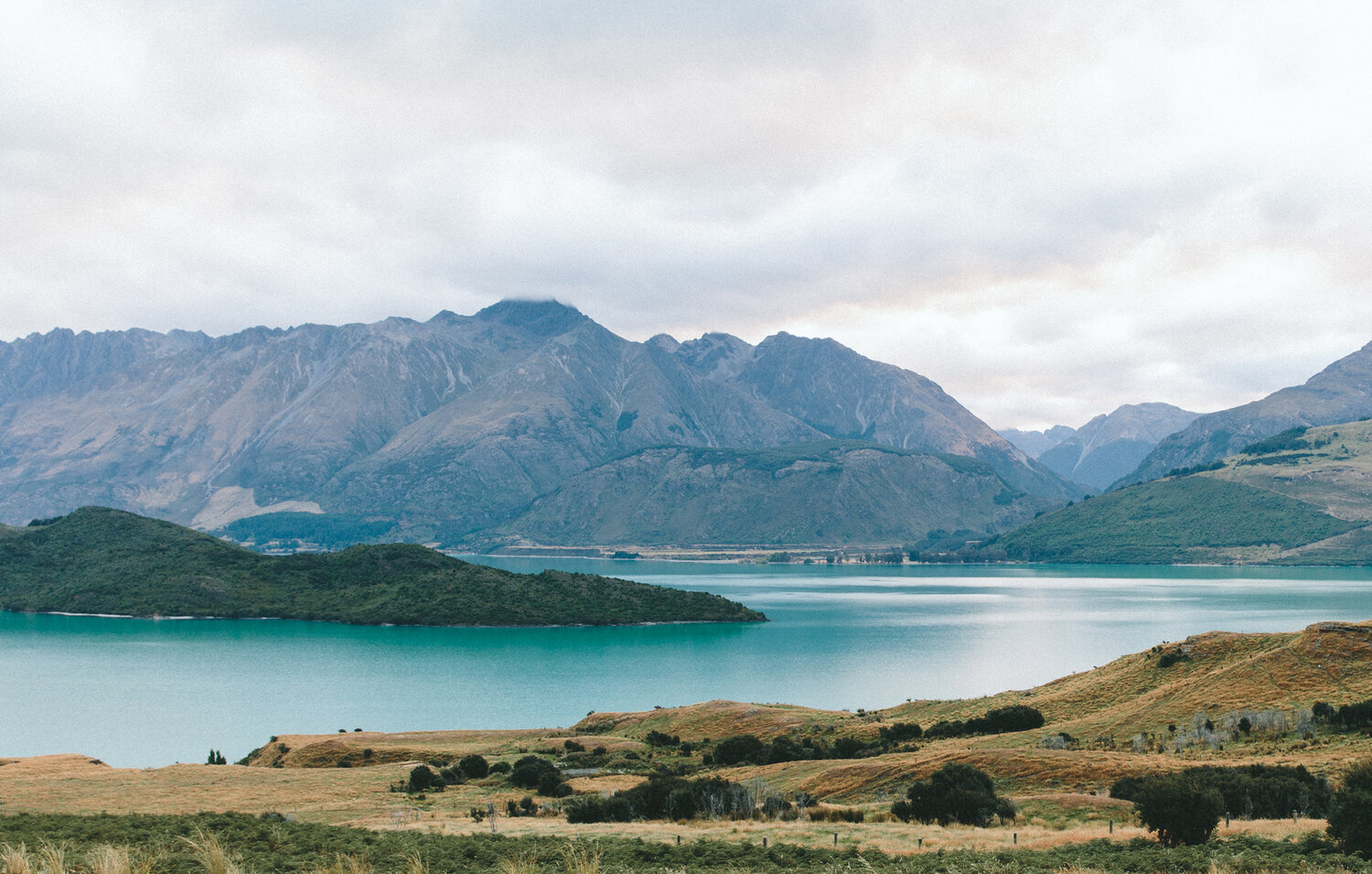
Empowering Innovation, Crafting the Future:
Together, we build next-generation AI systems for a brave new world.”
Optimize your operations with Brave AI Lab’s cutting-edge AI service trusted by over 10+ satisfied clients for innovation and efficiency.
contact@braveailab.com, social@braveailab.com
career@braveailab.com
Mon to Sat, 9.00 am to 7:00 pm